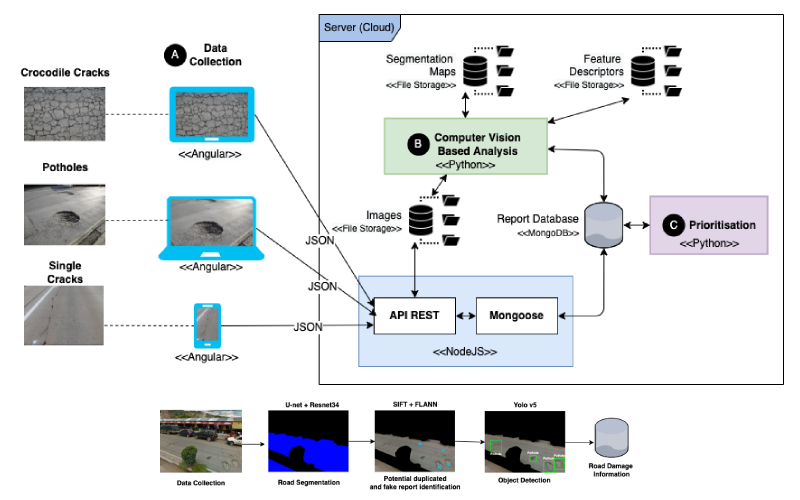
Journal of Sensor and Actuator Networks
Abstract: Maintenance of critical infrastructure is a costly necessity where developing countries often struggle to deliver timely repairs. The transport system acts as the arteries of any economy in development, and the formation of potholes on roads can lead to injuries and loss of lives. Recently, several countries have enabled pothole reporting platforms for their citizens, so that repair work data can be centralised and visible for everyone. Nevertheless, many of these have been interrupted because of the rapid growth of requests made by users. Not only these platforms failed to filter duplicate nor fake reports but also failed to classify their severity, albeit this information would be key in prioritising repair work and improving the safety of roads. In this work, we aim to develop a prioritisation system that combines deep learning models and traditional computer vision techniques to automate the analysis of road irregularities reported by citizens. The system consists of three main components. First, we propose a processing pipeline that segments road sections of repair requests with a U-net based model that integrates a pre-trained Resnet34 as the encoder. Second, we assess the performance of two object detection architectures -- EfficientDet and Yolov5 -- in the task of road damage localisation and classification. Two public datasets, the Indian Driving Dataset (IDD) and the Road Damage Detection Dataset (RDD2020), are preprocessed and augmented to train and evaluate our segmentation and damage detection models. Third, we apply feature extraction and feature matching to find possible duplicated reports. The combination of these three approaches allowed us to cluster reports according to their location and severity using clustering techniques. The results show that this approach is a promising direction for authorities to leverage limited road maintenance resources in an impactful and effective way.
BibTeX
author = { Edwin Salcedo and Mona Jaber and Jesús Requena Carrión },
title = { A Novel Road Maintenance Prioritisation System Based on Computer Vision and Crowdsourced Reporting },
journal = { Journal of Sensor and Actuator Networks },
year = { 2022 }
doi = { 10.3390/jsan11010015 }
url = { https://doi.org/10.3390/jsan11010015 }
volume = { 11 }
}