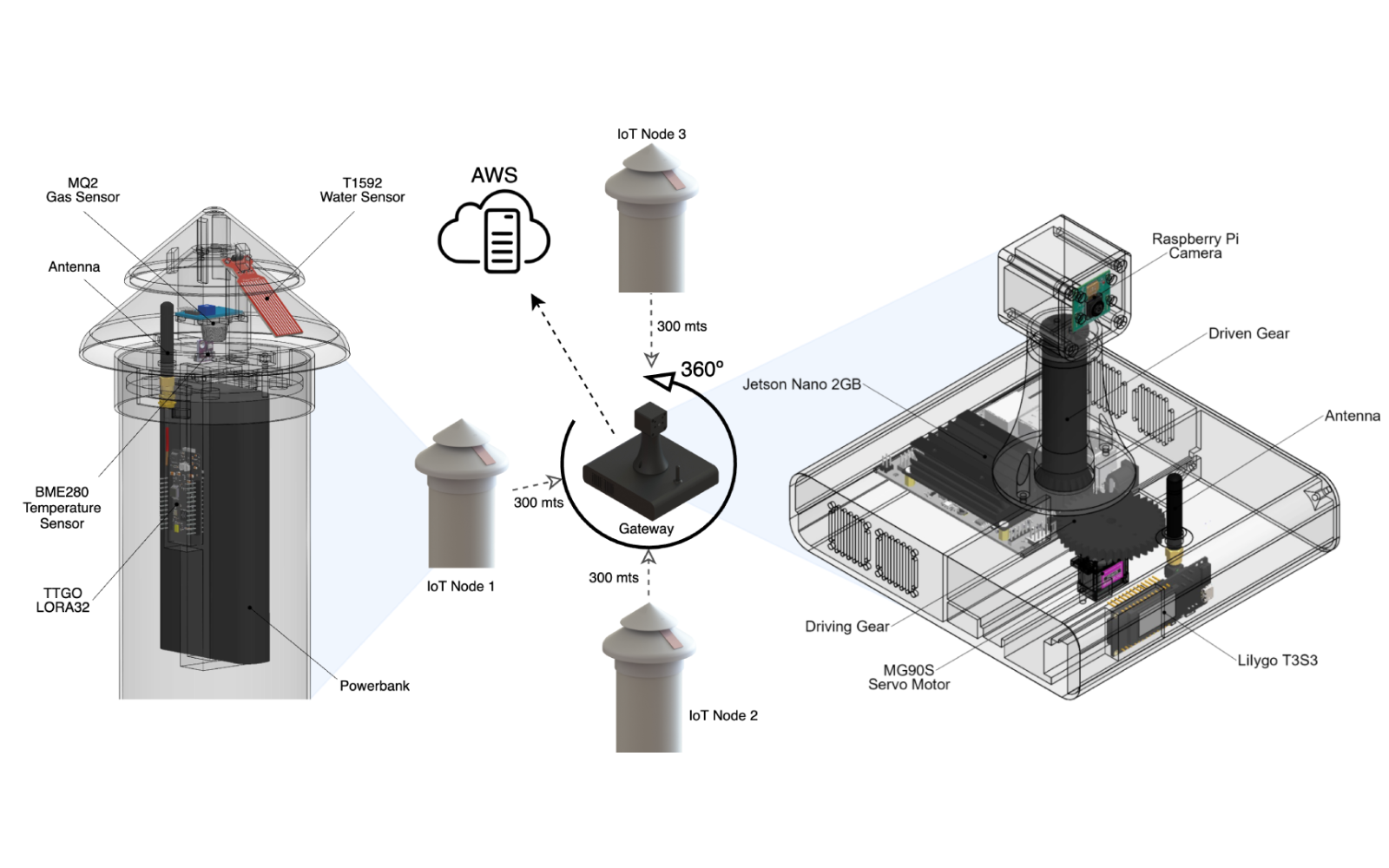
ICARA 2025, Zagreb, Croatia
Abstract: Early detection of forest fires is crucial to minimizing the environmental and socioeconomic damage they cause. Indeed, a fire's duration directly correlates with the difficulty and cost of extinguishing it. For instance, a fire burning for 1 minute might require 1 liter of water to extinguish, while a 2-minute fire could demand 100 liters, and a 10-minute fire might necessitate 1,000 liters. On the other hand, existing fire detection systems based on novel technologies (e.g., remote sensing, PTZ cameras, UAVs) are often expensive and require human intervention, making continuous monitoring of large areas impractical. To address this challenge, this work proposes a low-cost forest fire detection system that utilizes a central gateway device with computer vision capabilities to monitor a 360° field of view for smoke at long distances. A deep reinforcement learning agent enhances surveillance by dynamically controlling the camera's orientation, leveraging real-time sensor data (smoke levels, ambient temperature, and humidity) from distributed IoT devices. This approach enables automated wildfire monitoring across expansive areas while reducing false positives.
Paper and Supplementary Material
Latest version published on Feb. 12, 2025. Conference proceedings link
Author(s)
BibTeX
author = { Kenneth Bonilla Ormachea and Horacio Cuizaga and Edwin Salcedo and Sebastian Castro and Sergio Fernandez and Misael Mamani },
title = { ForestProtector: An IoT Architecture Integrating Machine Vision and Deep Reinforcement Learning for Efficient Wildfire Monitoring },
journal = { ICARA 2025 },
year = { 2025 }
}
Acknowledgements
This work was supported by a grant from Google AI, under the program TensorFlow Faculty Award 2021.